Harnessing the power of biology to create products of commercial and scientific value is a challenging problem. The goal of reducing biological engineering to a predictable discipline akin to mechanical or electrical engineering, while laudable, has met with limited success since many of the governing rules are still not known or well understood. Because fully engineering biological systems from first principles remains a distant dream, forward biological engineering will, for the foreseeable future, stay grounded in empirical methods requiring iterative design-create-test-learn (DCTL) cycles. The “create” part of DCTL refers to the transformational CRISPR-EnAbled Trackable genome Engineering (CREATE) technology that enables massively parallel genome editing.1
Sequence space is vast, and obtaining knowledge of where to intervene to achieve desired outcomes is a central challenge in engineering biological systems. The key to addressing this challenge is through large-scale diversity generation, where many thousands of individual ideas can be generated, realized, and evaluated in a rapid, efficient, and cost-effective manner. Ideas that show promise are then recombined in novel ways that leverage the principles of evolution (both natural and artificial) to efficiently navigate incomprehensibly large combinatorial sequence spaces.
This combinatorial search process is further accelerated through strategies that incorporate machine learning and exploit the information contained in empirically derived and prescriptive genotype-to-phenotype functional maps. Strategies and tools to carry out this approach to biological optimization at the protein level have been well developed over the last two decades and are now routinely used to create successful commercial processes and deliver new scientific insights.
In principle, the strategy for practical, generalized biological engineering at all scales (from gene to pathway to genome) has existed for some time. Yet implementation in practice had to await the development of technologies to rapidly and precisely edit the genome at all scales. Fortunately, the required technologies have recently become available through CRISPR-based genome editing. These technological breakthroughs now place us at the dawn of being able to engineer biological systems at previously unattainable scale and complexity. With the ability to efficiently intervene across the entire genome, in both massively parallel single and multiplexed combinatorial formats, researchers should be limited only by their imaginations and capacity to evaluate or characterize new ideas.
The wide availability of reliable, cost-effective strategies and tools for genome engineering will play a transformative role in biotechnology. The first step will be implementing novel approaches for forward engineering that incorporate CRISPR advances and solve the current scale limitations for genome editing pipelines by enabling the massively parallel generation of large numbers and varieties of edits, as well as highly multiplex combinatorial editing.
Empirical design
The DCTL cycle is a fundamental strategy for biological engineering. Its iterative approach is necessary because of the complexity of biology: the function of living systems cannot be predicted as easily as, say, an electrical circuit. This method effectively allows nature to be the guide, revealing at each step of the process the changes that improved or reduced fitness or increased or decreased the strength of a desired phenotype.
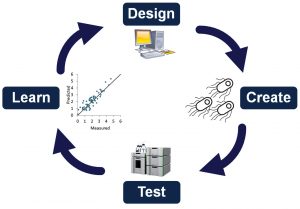
Even with a robust DCTL process, the ability to generate diversity remains critical for successful experimental outcomes. This requirement is true not only for areas well studied with forward engineering, such as single nucleotide polymorphisms (SNPs) and enzyme changes, but also for elements at the pathway or genome level. While there are examples of engineered SNPs, deletions, and amino acid changes, we are still limited in our ability to intervene on all the elements controlling biological systems. Future technologies that can deliver a combination of expression regulation, such as promoter or terminator changes, and protein coding edits would bring a step change in engineering biological systems.
DCTL begins with designing and building a diversity library that encompasses as many engineered changes as possible. These edits must then be tested individually across the library, at which point researchers can select the most promising candidates and further optimize results by recombining those changes.
When dealing with the limited scale of conventional editing and engineering technologies, biological engineers must recognize that screening even a fraction of a library can lead to significant improvements in outcomes. This is particularly true in multiplexed, combinatorial formats, where the power of recombination and selection over cycles of evolution has proven to be an exceedingly powerful search algorithm—for decades in the computer science and directed molecular evolution communities, for thousands of years in plant and animal domestication, and for billions of years by nature itself. The process eliminates changes that would be deleterious and reduces the space required for testing to only the changes most likely to be effective.
Scaling up
A new approach that has now been validated in several academic and bioindustrial laboratories significantly increases the scale at which CRISPR-enabled forward engineering experiments can be performed. The goal of this technique is to expand the number of edits and variety of edit types that can be done, such that scientists can obtain a far more comprehensive view of the universe of possible changes and effects to select the best ones more quickly. The increase in scale also enables multiplexed combinatorial editing on a level that is not feasible with conventional methods.
This technique involves high-throughput diversity generation, multiplexed combinatorial editing, genotyping, and phenotyping to allow scientists to build rich, prescriptive machine learning models that can be interrogated to formulate new, superior hypotheses to drive the next set of library designs.
In an early validation of the diversity generation aspect of this approach, scientists interested in improving lysine production created more than 16,000 edits across 19 genes using precise engineering, not random editing, in a process that took less than a month.1,2 This is in contrast to typical studies that target only a handful of locations in the genome over the course of months.
Another attempt at this experiment was later performed at even higher throughput, covering 200,000 edits. The results (not yet published) demonstrated that genome-wide approaches that include knockouts, upregulation, and downregulation of genes—many of which are outside the core pathway for lysine—are all valuable sources of beneficial diversity for forward engineering of genomes.
In another pilot project, researchers recapitulated a project that had used random mutagenesis to improve the thermotolerance of Escherichia coli and identified nearly 650 SNPs present in the evolved cell populations.3 The new version of this project incorporated the original set of SNPs, plus 52,000 additional edits.1 The work confirmed the original results and also found other edits relevant to the phenotype of interest. In fact, the single most effective edit was a two-base change, which could not have been detected using the original adaptive evolution approach.
Looking ahead
Existing methods for CRISPR-based editing and forward engineering, in general, are limited by throughput, editing ability, or cost. There is a pressing need for affordable, high-throughput alternatives that would enable multibase edits and rapid multiplexed combinatorial editing to accelerate progress in genome engineering.
Importantly, this kind of approach would make it possible for even small laboratories to get involved in genome engineering; today, the laborious steps associated with CRISPR editing can prevent such labs from taking part. Ultimately, by generating far more data about the fitness effects of programmed genomic variation on various organisms and their phenotypes of interest, fields such as synthetic biology, agricultural biology, and even healthcare will see real innovation.
References
1. Garst AD, Bassalo MC, Pines G, et al. Genome-wide mapping of mutations at
single-nucleotide resolution for protein, metabolic and genome engineering.
Nat. Biotechnol. 2016, 35: 48–55. DOI: 10.1038/nbt.3718.
2. Bassalo MC, Garst AD, Choudhury A, et al. Deep scanning lysine metabolism in Escherichia coli. Mol. Syst. Biol. 2018; 14: e8371. DOI: 10.15252/msb.20188371.
3. Tenaillon O, Rodríguez-Verdugo A, Gaut RL, et al. The molecular diversity
of adaptive convergence. Science 2012; 335: 457–461. DOI: 10.1126/
science.1212986.
Richard Fox, PhD, is executive director of data science at Inscripta.