
Head of Science Analytics
Charles River Laboratories
Picture a world where our genomes are sequenced at birth, genetic errors corrected, and continuous monitoring of vital signs allows for tailored dietary and lifestyle adjustments to preempt diseases. Should illness arise, personalized drugs, precisely crafted to meet an individual’s unique needs, physiology, and phenotypes, would be deployed.
This may sound like a page from a science fiction novel, yet certain hard facts suggest otherwise:
- The cost of full genome sequencing has plummeted from $2.7 billion in the early 2000s to under $1,000 today,1 paving the way for genome sequencing to become as routine as standard newborn blood screenings.
- In vivo base editing via infusion can
effectively reduce high cholesterol levels,2 marking a significant leap toward safe and targeted gene therapy. - Despite the ethical controversies surrounding Theranos,3 the concept of regular blood biomarker monitoring persists. Daniel Ek, Spotify’s CEO, launched Neko Health, promising artificial intelligence (AI)-backed full-body scans to aid in the early detection of skin conditions, cancer, cardiovascular diseases, and more.4
- The Personalized Medicine Coalition reports that in 2022, personalized medicines constituted 34% of all new drug approvals by the U.S. Food and Drug Administration.5
- Prominent technology entrepreneurs such as Bryan Johnson are engaging in a “Rejuvenation Olympics,” vying to decelerate their biological aging.6
These advancements are intrinsically linked to significant technological progress, from increased computing power to enhanced data storage capabilities, propelling AI to the forefront of today’s reality.
Assessing the clinical progress of AI-designed drugs
AI-designed drugs have already progressed to clinical trials.7 Their rapid advancement through discovery and preclinical stages is particularly striking. However, the true test for AI-designed drugs lies in their clinical performance, which, to date, has been underwhelming. In 2022, BenevolentAI’s drug for atopic dermatitis failed to outperform a placebo in a Phase IIa trial,8 and Exscientia’s cancer drug candidate EXS-21546 fell short in early clinical phases this year.9
We must remember, however, that countless AI-generated drugs in preclinical stages haven’t yet reached clinical testing. Drawing conclusions from a limited set of examples is premature. Traditional drug development methods might have led to similar outcomes, but AI’s advantage lies in identifying potential failures earlier and more cost-effectively.

Reimagining drug R&D with the help of data and technology
For many years, the pharmaceutical sector treated data as expendable, focusing solely on wet lab experiments to answer scientific questions, and often disregarding the data once the query was resolved. Only recently has the industry begun to recognize the value of this data, seeing it as a potential gold mine. However, extracting valuable insights from this computational ore has proven challenging.
There’s a noticeable cultural shift within the industry as companies start to see themselves as data companies driving a transformation that emphasizes the reuse of diverse and historical data to shape predictions and inform decision-making.10
Digital-native pharmaceutical firms, often referred to as techbio firms, attribute their early successes to several factors, the most crucial being their inception as technology companies without the burden of legacy data or technology. Techbio firms are advantaged because they lack data silos and have data architectures designed to handle massive data volumes and run data-intensive applications.
Starting in silico—but then seeking proof in the wet lab
The conventional drug R&D process, while well established, is fraught with challenges. It typically involves selecting a target in the body, designing a molecule to interact with it, synthesizing the molecule, and then testing its safety and efficacy. This method is notably slow and costly and has a high failure rate. Many compounds that show promise in the lab often fail during clinical trials due to efficacy or safety issues. AI is redefining this process by addressing critical failure points such as helping scientists in selecting the right target and designing safe and effective molecules.11
In target identification, AI is playing an increasingly vital role. The sheer volume of scientific literature is beyond human capacity to fully comprehend, but AI can mine extensive scientific databases. It extracts and analyzes data to predict promising drug targets, uncovering connections that might elude human researchers.
AI is also revolutionizing the design of drug molecules. The largest compound libraries for high-throughput screening contain, at most, around 10 million compounds. This pales in comparison to the estimated 1033 possible drug-like compounds.12 Given the complex nature of molecular interactions within the human body, AI’s proficiency in sifting through countless possibilities to identify the most promising molecules is invaluable.11
However, it’s important to recognize that AI is not a panacea. The validation of drug efficacy and safety still relies on traditional experiments and human clinical trials. The role of AI in drug development is not to replace these fundamental steps but to enhance the efficiency and focus of the drug R&D process. Where numerous experiments might have been necessary previously, AI can guide researchers to the most promising experiments, significantly cutting down the time and cost involved.11
Impacting the (dreadful) pharma metrics
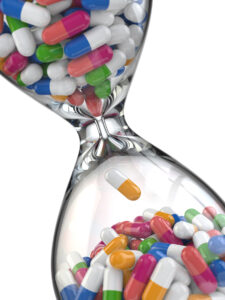
Launching a drug is often likened to a Sisyphean undertaking,13 yet the potential of AI in accelerating drug R&D makes the significant investments and digital transformation efforts in the pharmaceutical industry seem worthwhile. In an industry where spending over $6.5 billion, failing 95% of the time, and taking an average of 12 years to release a single drug is the norm, the promise of AI is particularly compelling.12 Initial forays into AI-assisted drug development have shown that it’s possible to cut development time by a factor of 15 and to reduce costs by 70% of what they would be without AI.24
An additional, crucial benefit of AI in drug development is the reduction of animal use in preclinical research. The research community is dedicated to achieving the 3Rs (Replacement, Reduction, and Refinement in the use of animals). Virtual Control Groups have already been shown to potentially reduce animal use by 25% by substituting control group animals with preexisting randomized data sets.14
Finally, AI’s ability to invent entirely novel small-molecule drugs11 and create new proteins with specific therapeutic properties is nothing short of revolutionary.15 AI-generated proteins surpass the limitations of 3 billion years of natural evolution, showcasing the boundless potential that generative AI offers drug discovery.15
Navigating the hype—and reality—of AI in pharma
AI, while transformative, is not a silver bullet but a significant addition to the pharmaceutical industry’s toolkit. It’s crucial to balance hype with realistic expectations and learning from past experiences.
IBM Watson’s healthcare journey exemplifies this cautionary tale. Lauded for its potential to revolutionize oncology care with advanced insights and patient care, the reality fell short. Winning at “Jeopardy!” is a far cry from matching the right patients to clinical trials or predicting cancer progression and treatment outcomes.16
Novartis faced similar challenges with its ambitious Data42 project. Positioned as a major digital and data initiative, the goal was to mine 2 million patient-years of data for novel drug–disease correlations.17 However, the project faced scale-backs after substantial investment due to cost-cutting measures and complexities in achieving its vision.18,19
In contrast, Google DeepMind represents a successful integration of AI into life sciences with AlphaFold. This AI system can accurately predict protein structures, a task traditionally demanding extensive, time-consuming experiments like X-ray protein crystallography. AlphaFold’s ability to predict over 200 million protein structures, using existing data from the Protein Data Bank, has significant implications for structure-based drug design and showcases the potential of AI when applied effectively.20,21
Charting a future path in AI-driven drug R&D
As we envision the future of medicine, reshaped by data and digital technologies, we’re not just contemplating a shift in methods, we’re foreseeing a transformation that benefits the entire healthcare ecosystem. Pharmaceutical companies will optimize drug R&D processes, reducing risks, costs, and animal testing requirements while accelerating market entry. The critical question now is not whether to adopt data and AI-driven R&D—that’s unequivocally beneficial—but rather how to implement it effectively while navigating the potential pitfalls encountered when transitioning from traditional to digital-first R&D models.
Key steps for organizations to succeed in this transition include:
1. Cultivating a data-centric organizational culture: Overcoming the misconception that AI will replace jobs is crucial. In the pharmaceutical industry, AI augments rather than replaces human expertise. This is also the case in other fields. In pathology, the traditional role of practitioners is expected to be enhanced by AI—even though the best practitioners are sometimes outperformed by the technology.22,23
2. Adapting business models to the data-driven era: Pharmaceutical companies might need to forge new partnerships for data access or technology acquisition. Contract research organizations should consider evolving their business models to accommodate AI-led programs, potentially shifting from traditional fee structures to milestone-based agreements, thereby reducing engagement risks.24,25
3. Establishing a circular data economy: Emphasizing the non-disposability of data and advocating for their reuse creates a virtuous cycle. Ensuring that all data adhere to FAIR principles (that is, to findability, accessibility, interoperability, and reusability) is a fundamental part of this approach.
Finally, the potential of generative AI cannot be overlooked. The ability to design novel proteins and structures, for scientists without data science expertise, is poised to revolutionize drug R&D.15 The challenge lies not just in the technology itself, but in ensuring its widespread adoption and trust within the scientific community. Although this journey is bound to be tortuous, it is well to start with a decisive stride toward a future where AI and human intelligence synergize to redefine healthcare.
Thibault Géoui, PhD, is head of science analytics at Charles River Laboratories.
References
- Jennings K. How Human Genome Sequencing Went From $1 Billion A Pop To Under $1,000. Forbes. October 28, 2020.
- Kaiser J. Base editing, a new form of gene therapy, sharply lowers bad cholesterol in clinical trial. Science. November 12, 2023.
- Carreyrou J. Bad Blood: Secrets and Lies in a Silicon Valley Startup. Picador; 2018.
- Sawers P. Daniel Ek’s Neko Health raises $65M for preventative healthcare through full-body scans. TechCrunch. July 5, 2023.
- Personalized Medicine Coalition. A New Vision for Health.
- Kim W. Inside the very strange, very expensive race to “de-age”. Vox. September 26, 2023.
- Arnold C. Inside the nascent industry of AI-designed drugs. Nat. Med. 2023; 29: 1292–1295.
- Matsuyama K. (2023, Nov 13). Race for First Drug Discovered by AI Nears Key Milestone. Bloomberg. November 13, 2023.
- Armstrong A. AI drug hunter Exscientia chops down ‘rapidly emerging pipeline’ to focus on 2 main oncology programs. Fierce Biotech. October 3, 2023.
- Ferrero E, Brachat S, Jenkins JL, et al. Ten simple rules to power drug discovery with data science. PLoS Comput. Biol. 2020; 16(8): e1008126. DOI: 10.1371/journal.pcbi.1008126.
- Heaven WD. AI is dreaming up drugs that no one has ever seen. Now we’ve got to see if they work. MIT Technology Review. February 15, 2023.
- Polishchuk PG, Madzhidov TI, Varnek A. Estimation of the size of drug-like chemical space based on GDB-17 data. J. Comput. Aided Mol. Des. 2013; 27: 675–679. DOI: 10.1007/s10822-013-9672-4.
- Schuhmacher A, Hinder M, von Stegmann Und Stein A, et al. Analysis of pharma R&D productivity—a new perspective needed. Drug Discov. Today 2023; 28(10): 103726. DOI: 10.1016/j.drudis.2023.103726.
- Steger-Hartmann T, Kreuchwig A, Vaas L, et al. Introducing the concept of virtual control groups into preclinical toxicology testing. ALTEX 2020; 37(3): 343–349. DOI: 10.14573/altex.2001311.
- Ingraham JB, Baranov M, Costello Z, et al. Illuminating protein space with a programmable generative model. Nature 2023; 623: 1070–1078. DOI: 10.1038/s41586-023-06728-8.
- O’Leary L. How IBM’s Watson Went from the Future of Health Care to Sold Off for Parts. Slate. January 31, 2022.
- Mijuk G. The data42 program shows Novartis’ intent to go big on data and digital. Novartis. March 11, 2020.
- Alich H. Novartis is cutting back on its AI project to save money. Handelszeitung. August 7, 2023.
- Michel, S. Novartis scales back AI project Data42.
- Silver D, Huang A, Maddison C, et al. Mastering the game of Go with deep neural networks and tree search. Nature 2016; 529: 484–489. DOI: 10.1038/nature16961.
- Heaven WD. This is the reason Demis Hassabis started DeepMind. MIT Technology Review. February 23, 2022.
- Miller F. Will AI take my job? The Times. November 8, 2023.
- 23.Baxi V, Edwards R, Montalto M, et al. Digital pathology and artificial intelligence in translational medicine and clinical practice. Mod. Pathol. 2022; 35: 23–32. DOI: 10.1038/s41379-021-00919-2.
- Goodier C. Accelerating drug discovery & development. Deloitte. https://www2.deloitte.com/uk/en/pages/deloitte-analytics/articles/accelerating-drug-discovery-and-development.html
- Logica.