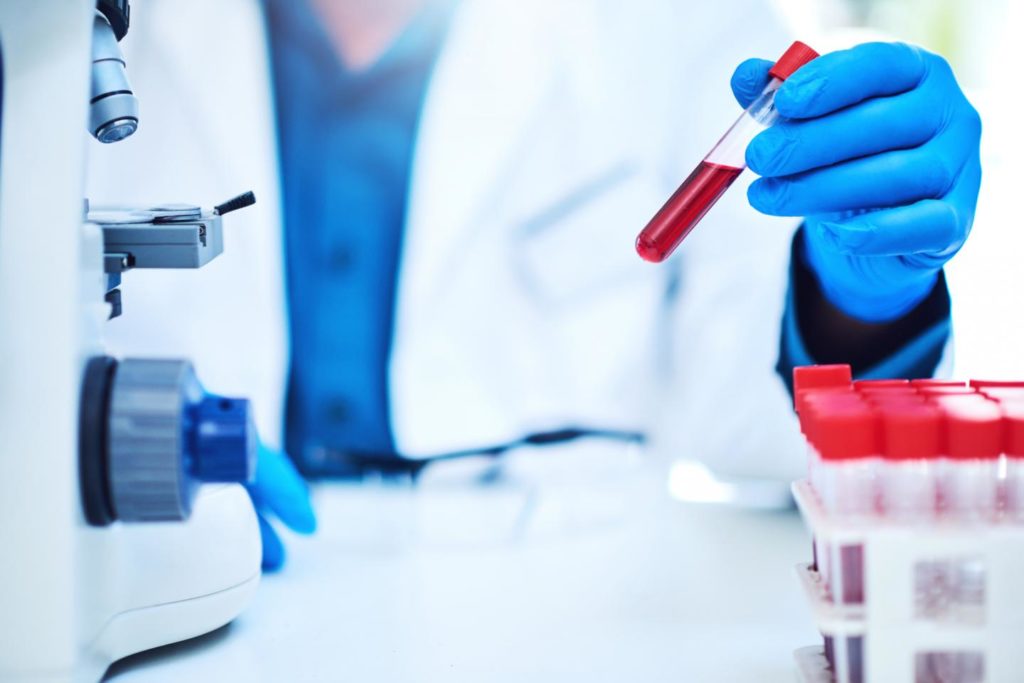
A multidisciplinary team at the University of Pennsylvania has used machine learning to develop a liquid biopsy that can detect early stages of the most common form of pancreatic cancer by measuring multiple biomarkers in a single blood sample. In a blinded study, the multi-analyte test was found to be more accurate than single biomarker analysis at detecting pancreatic ductal adenocarcinoma (PDAC), and was also better at staging disease than stand-alone imaging.
“Although a larger validation study is needed, this test may improve early disease detection and, when performed in addition to diagnostic imaging, patient selection for curative intent surgery,” the investigators concluded in their published paper in Clinical Cancer Research, which is titled, “A multi-analyte panel consisting of extracellular vesicle miRNAs and mRNA, cfDNA, and CA19-9 shows utility for diagnosis and staging of pancreatic cancer.” The research team included scientists at the Perelman School of Medicine, the Abramson Cancer Center, and the School of Engineering and Applied Science.
PDAC is the third leading cause of cancer deaths in the United States, with an overall five-year survival rate of 9%. Cases are commonly not diagnosed until the disease has already spread, and most patients survive for less than one year following their diagnosis.
If the disease is caught early, patients may be candidates for surgery to remove the cancer, which can be curative. For patients with locally advanced disease that has not spread beyond the pancreas, but who are not candidates for surgery based on the size or location of the tumor, treatment involves three months of systemic therapy like chemo- or radiation therapy, then reassessment to see if surgery is an option. However, for patients whose disease has spread there are currently no curative treatment options.
Diagnosis and staging of PDAC rely on techniques including biopsy, computerized tomography (CT), and magnetic resonance imaging, but distinguishing patients with localized disease from those with metastases remains challenging because standard of care imaging techniques may miss occult metastases. “… even for patients with pathologically confirmed PDAC, standard of care imaging can have low sensitivity to detect early metastatic disease,” the authors wrote. “This complicates disease staging and therapy selection, including curative-intent surgery.”
“Right now, the majority of patients who are diagnosed already have metastatic disease, so there is a critical need for a test that can not only detect the disease earlier but also accurately tell us who might be at a point where we can direct them to a potentially curative treatment,” said the study’s co-senior author Erica Carpenter, PhD, director of the Liquid Biopsy Laboratory and a research assistant professor of medicine. Carpenter co-led the study with David Issadore, PhD, an associate professor of bioengineering and electrical and systems engineering.
Various blood-based liquid biopsy markers have been developed to try to address these diagnostic challenges, but they have shown low sensitivity for detecting early-stage disease, the authors continued. And while the team’s previous work in a mouse model of pancreatic cancer had shown that analyzing tumor-associated extracellular vesicles can help to detect early-stage disease, they acknowledged that the accuracy of this approach in detecting early disease in human patients hasn’t yet been demonstrated.
The team now reports on their development and assessment of a panel of PDAC diagnostic biomarkers, including tumor-associated extracellular vesicle miRNA, mRNA, circulating cell-free DNA, and carbohydrate antigen 19-9 (CA19-9), which they claim can accurately identify patients with PDAC, and also improve the detection of occult metastases that might be missed by initial imaging.
Their work harnessed machine learning to analyze data from the selected panel of biomarkers. As they explained, “To generate a predictive panel of biomarkers, each biomarker needs predictive power and the constituent biomarkers should not correlate with one another, such that each biomarker carries some unique information about the state of the patient.” Using the panel of 14 biomarkers, they trained the machine learning model with a set of 15 healthy controls, 12 disease controls (3 intraductal papillary mucinous neoplasm, and 9 pancreatitis), and 20 patients with PDAC of various stages.” In this blinded assessment the test was 92% accurate in its ability to detect disease, which outperforms the 89% accuracy of measuring CA19-9 alone, they noted. The assay also demonstrated 84% accuracy in determining disease staging, which was an improvement over the 64% accuracy exhibited by imaging alone.
The authors acknowledged that their test still needs to be validated in a larger cohort, but say it could represent a major advance in PDAC diagnosis and help to identify patients who are eligible for potentially curative surgery. “Here we describe a multi-analyte liquid biopsy to better detect and stage PDAC from a single blood sample,” they wrote. “This approach was able to distinguish patients with PDAC from those without. Moreover, among patients with PDAC, the model could improve detection of occult metastatic disease that was imaging-negative at baseline and only discovered intraoperatively or by subsequent imaging within four months of baseline blood draw.”
Carpenter further stated, “If validated, this test could not only provide a key tool for at-risk patients, but also a monitoring tool for patients with certain known risk factors like BRCA mutations.”