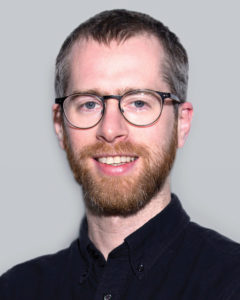
Chief Technical Officer
PhoreMost
Drug discovery and development is often plagued by candidate attrition and late-phase failure. Screening plays a key role in target identification and validation, and so the quality of the chosen screening technique can be a deciding factor in whether a new drug makes it into the clinic. Gene editing technologies such as CRISPR, or gene knockdown technologies such as RNAi, have yielded positive results, but there is a risk that loss-of-function screening tools cannot analyze the more subtle biological processes that contribute to clinical response. Biotech and pharma companies are therefore now looking to new, multimodal screening approaches to identify novel drug-target interaction candidates.
Traditional target discovery methods have led to a reliance on a very small proportion of the genome.1 Less than 1% of the proteome has been successfully targeted for therapeutic intervention, and some of these biomolecules, such as kinases, ion channels, and G protein–coupled receptors, are being vastly overrepresented.2 It is, therefore, clear that we need to develop unbiased tools and methods to fully explore new target spaces.
RNA interference (RNAi) was at one point considered the method of choice for target validation in cell culture systems. However, despite its potential and the significant resources put in place to support RNA-based pooled screening, promising candidates have failed to contribute toward clinical drug development. Later reports have linked the technology to off-target effects, incorrect results, and a lack of reproducibility.3 As a result, RNAi-generated data is neither clean nor robust enough to be reliably adopted by the industry,4 and although screens using higher numbers of replicates and short hairpin RNAs (shRNAs) have shown more promising results, there is still much room for improvement.5,6
CRISPR screening has proven to be a more favorable target identification technique. The technical advantages for detection and statistical significance, coupled with high effect rate through genetic ablation, allow us to observe meaningful phenotypic effects, increasing the efficiency compared to shRNA. However, CRISPR screening is still not the perfect answer. Slight differences in the experimental approach or in the libraries of guide RNAs used can lead to restricted hit overlap and incomplete relative ranking between apparently identical parallel studies.7,8 This demonstrates the importance of careful experimental validation when relying on a single screening tool to populate development pipelines with novel drug-target interaction candidates.
Aside from the challenges that different screening approaches face, the resulting hits also do not guarantee success. A small molecule inhibitor can rarely achieve 100% loss of function, and gene knockout screening may result in a pool of unactionable targets for drug development as it yields a theoretical maximum effect size. One promising way around this is to conduct gene silencing and gene editing screening in parallel, to skew the hit nomination in favor of targets that are robustly discovered even with modest loss of function.8 However, gene editing and silencing can be blunt instruments and do not allow adequate interrogation of more complex biological interactions.
With these limitations in mind, researchers are now turning to a multimodal approach to augment the current drug discovery landscape, and this has led to some promising new innovations. An example of this is PROTEINi®, a protein interference technique that has been developed by PhoreMost. PROTEINi can explore and identify drug-mimetics in live cells at a vast scale using a phenotypic readout.
An important feature of PROTEINi is that it can amplify the existing target identification space by identifying proteins and complexes that would normally be considered undruggable. Since every responding therapeutic node can be probed by PROTEINi, the technology allows researchers to examine the biological activity that modulates regulatory pathways in more depth, giving more insight than simple loss-of-function studies. Tools such as this could unlock alternative, direct routes to drug development through systematically scanning the entire proteome to identify the best new targets for drug development and, crucially, determine how to drug them.
Another important aspect of the drug discovery process is developing robust cell models—especially vital when carrying out in vitro screening. This could be a simple cell line–based model, or a more complex physiological model, depending on the requirements of the experiment.
Those working in cancer target discovery are fortunate to have an abundance of accessible cell panels at their disposal. However, there are some concerns around hit quality due to conflating mutations and complex genomic and epigenomic landscapes. Isogenic systems can offer a cleaner background for screening, but it is difficult to replicate these results in patient models, and so screening in multiple cell backgrounds simultaneously offers the best option for reliable and translatable hit discovery.
In other therapeutic areas, the necessary screen-ready physiological cell models are only now becoming available to researchers. The discovery and improved biomanufacturing of induced pluripotent stem cells (iPSCs) have been pivotal to the improvement of cell models. However, it may still require a more complex phenotypic analysis of perturbation response to unlock their full potential in target discovery.
Single-cell analysis is able to provide a crucial additional degree of resolution in some forms, although this is at a smaller scale than ideally required.9–11 So here, too, it is clear that multiple models and cell systems will be required to properly assess drug target potential.
Historically, newly identified targets have been assessed and prioritized based on their druggability. As a result, a significant number of targets have been discarded, wasting the time and effort that went into finding them. Drug-mimetic screening, such as with PROTEINi, gives the opportunity to capture targets that have been preselected for ligandability. New tools and systems not only challenge traditional target identification rules, they also allow screening to be conducted in an unconstrained manner.
Overall, each screening tool discussed has advantages and applications within select therapeutic spaces. Therefore, by maximizing the respective benefits of each method, multimodel screening presents itself as the most effective approach to progress from target identification to new medicines. This multimodal approach to screening has the capacity to capture the total target space, bringing better drugs to the market, faster.
Benedict C.S. Cross, PhD, is chief technical officer at PhoreMost.
References
1. Terry C, Lesser N. Unlocking R&D productivity: Measuring the return from pharmaceutical innovation 2018. London: Deloitte Centre for Health Solutions; 2018. Accessed: July 30, 2020.
2. Santos R, Urso O, Gaulton A, et al. A comprehensive map of molecular drug targets. Nat. Rev. Drug Discov. 2016; 16: 19–34.
3. Offord C. RNAi’s future in drug-target screening. The Scientist. Published: April 16, 2017. Accessed: July 30, 2020.
4. Bhinder B, Djaballah H. A decade of RNAi screening: Too much hay and very few needles. Drug Discovery World. Published: 2013. Accessed: July 30, 2020.
5. Kampmann M, Bassik MC, Weissman JS. Integrated platform for genome-wide screening and construction of high-density genetic interaction maps in mammalian cells. Proc. Natl. Acad. Sci. USA 2013; 110(25): E2317–E2326). DOI:10.1073/pnas.1307002110.
6. Morgens DW, Deans RM, Li A, Bassik MC. Systematic comparison of CRISPR/Cas9 and RNAi screens for essential genes. Nat. Biotechnol. 2016; 34: 634–636.
7. Shalem O, Sanjana NE, Hartenian E, et al. Genome-scale CRISPR-Cas9 knockout screening in human cells. Science 2014; 343: 84–87.
8. Le Sage C, Lawo S, Panicker P, et al. Dual direction CRISPR transcriptional regulation screening uncovers gene networks driving drug resistance. Sci. Rep. 2017; 7: 17693.
9. Datlinger P, Rendeiro AF, Schmidl C, et al. Pooled CRISPR screening with single-cell transcriptome readout. Nat. Methods 2017; 14: 297–301.
10. Dixit A, Parnas O, Li B, et al. Perturb-Seq: Dissecting Molecular Circuits with Scalable Single-Cell RNA Profiling of Pooled Genetic Screens. Cell 2016; 167: 1853–1866 e17
11. Adamson B, Norman TM, Jost M, et al. A Multiplexed Single-Cell CRISPR Screening Platform Enables Systematic Dissection of the Unfolded Protein
Response. Cell 2016; 167: 1867–1882 e21.